VALSE 2021
第一次参加学术会议,记录一下在杭州的收获、和点滴。
不积小流无以成江海。
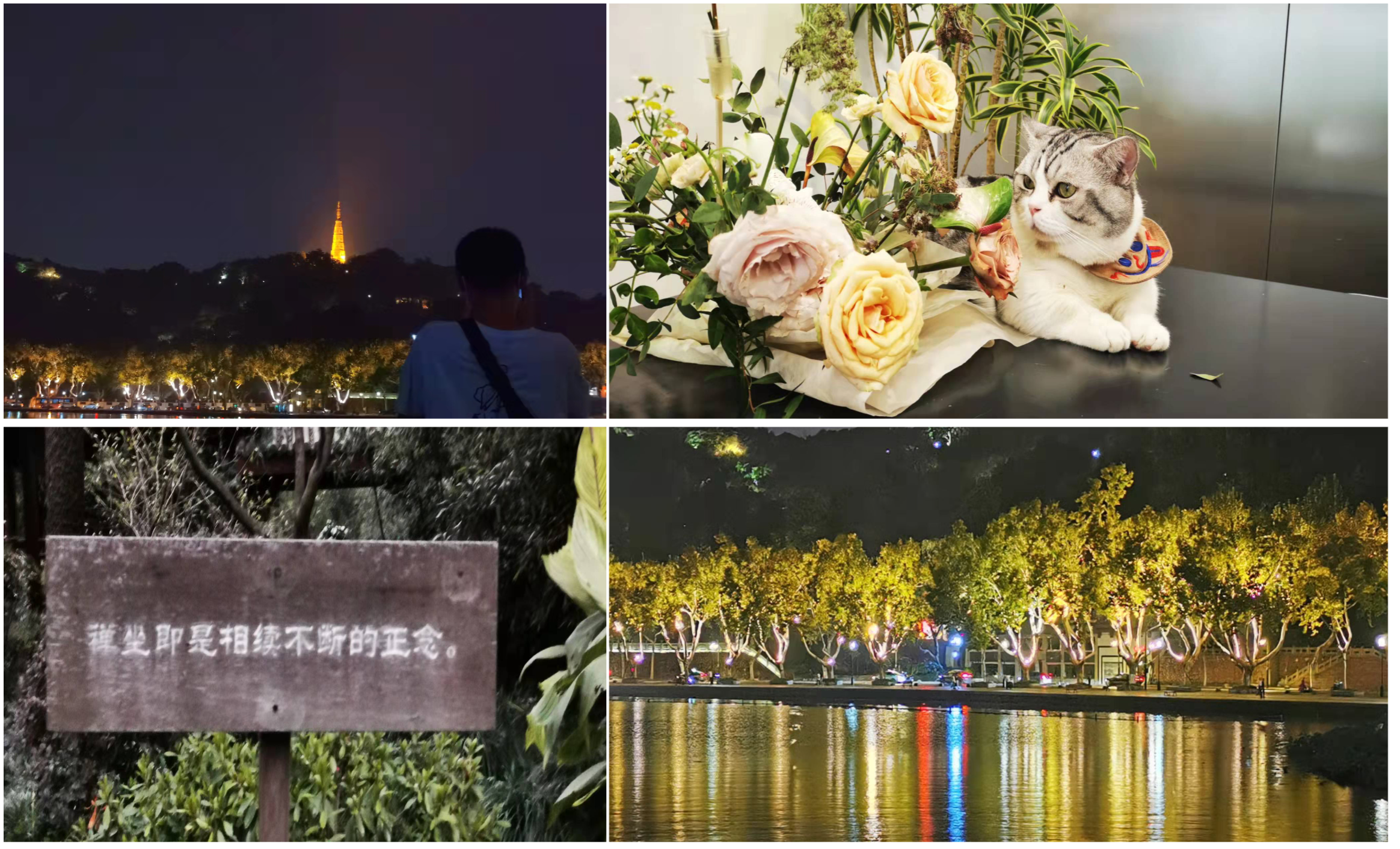
时间:10.08
如何突破机器学习的先验假设
徐院士主要介绍了机器学习在使用是的五个先觉条件,这也是限制机器学习的五个方面的假设。在研究机器学习、深度学习的任务中时,可从这五个方面着手,科学地(数学地)进行研究设计。
这五个先觉条件,个人认为是机器学习算法设计的五个方面(损失函数、模型结构/正则项涉及、数据分布、收敛性)。在数学的层面对其分析,对研究会更有帮助。
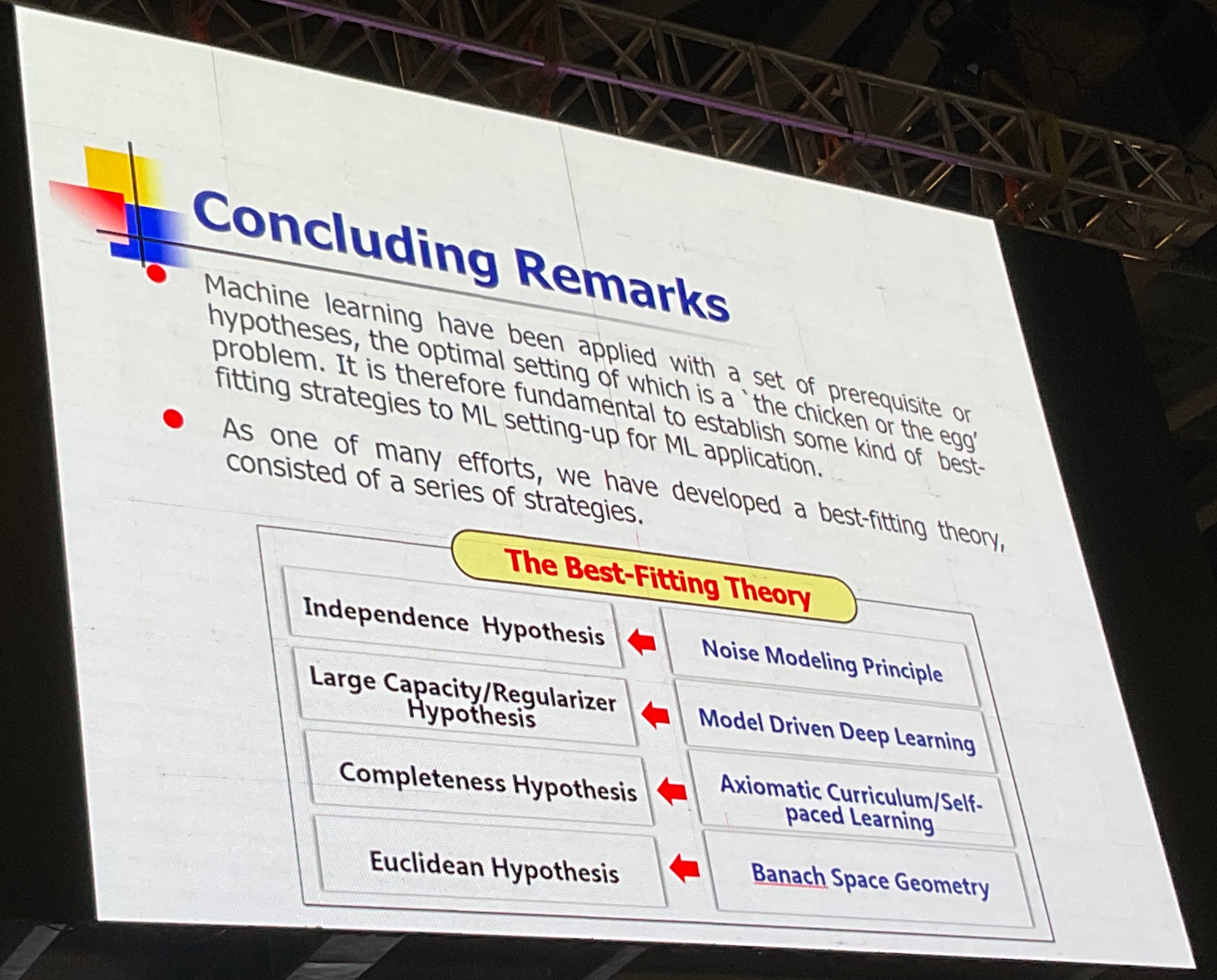
- Independence Hypothesis (独立假设)
- Large Capacity/Regularizer hypothesis(大容量假设/正则项假设)
- Completeness Hypothesis(训练数据完备性假设)
- Euclidean Hypothesis(欧式空间假设)
CSDN上有完整的报告内容
参考文献:
![]() |
![]() |
![]() |
![]() |
时间:10.08
动态神经网络综述
动态网络最新综述:Dynamic Neural Networks: A Survey [文献地址]
![]() |
![]() |
时间:10.08
AI赋能高分遥感目标检测与识别:挑战、对策及展望
![]() |
![]() |
1.旋转不变形
Cheng G, Zhou P, Han J. Learning rotation-invariant convolutional neural networks for object detection in VHR optical remote sensing images[J]. IEEE Transactions on Geoscience and Remote Sensing, 2016, 54(12): 7405-7415.
Cheng G, Han J, Zhou P, et al. Learning rotation-invariant and fisher discriminative convolutional neural networks for object detection[J]. IEEE Transactions on Image Processing, 2018, 28(1): 265-278.
2.有向目标检测
Xie X, Cheng G, Wang J, et al. Oriented R-CNN for Object Detection[C]//Proceedings of the IEEE/CVF International Conference on Computer Vision. 2021: 3520-3529.
Cheng G, Wang J, Li K, et al. Anchor-free Oriented Proposal Generator for Object Detection[J]. arXiv preprint arXiv:2110.01931, 2021.
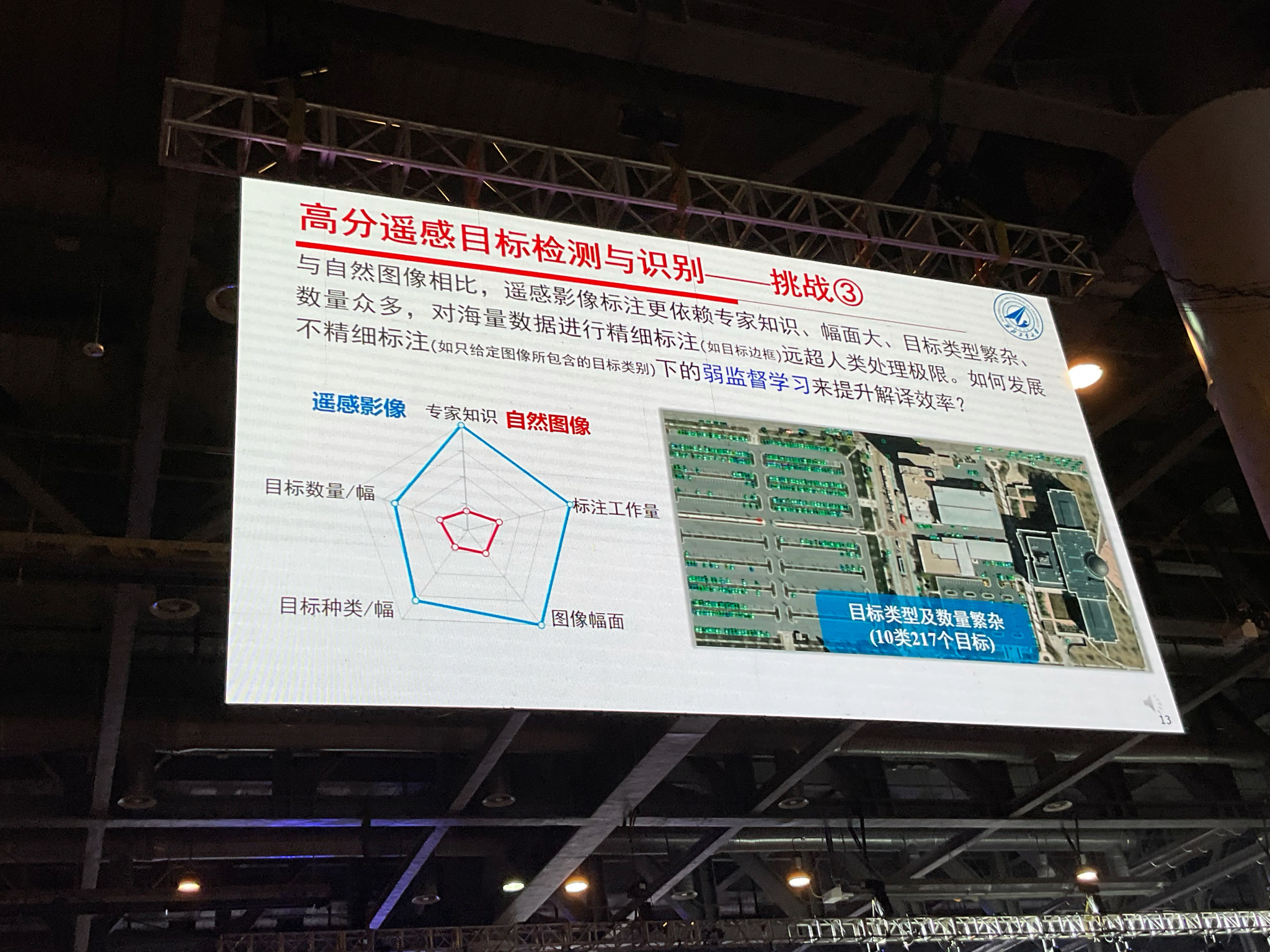
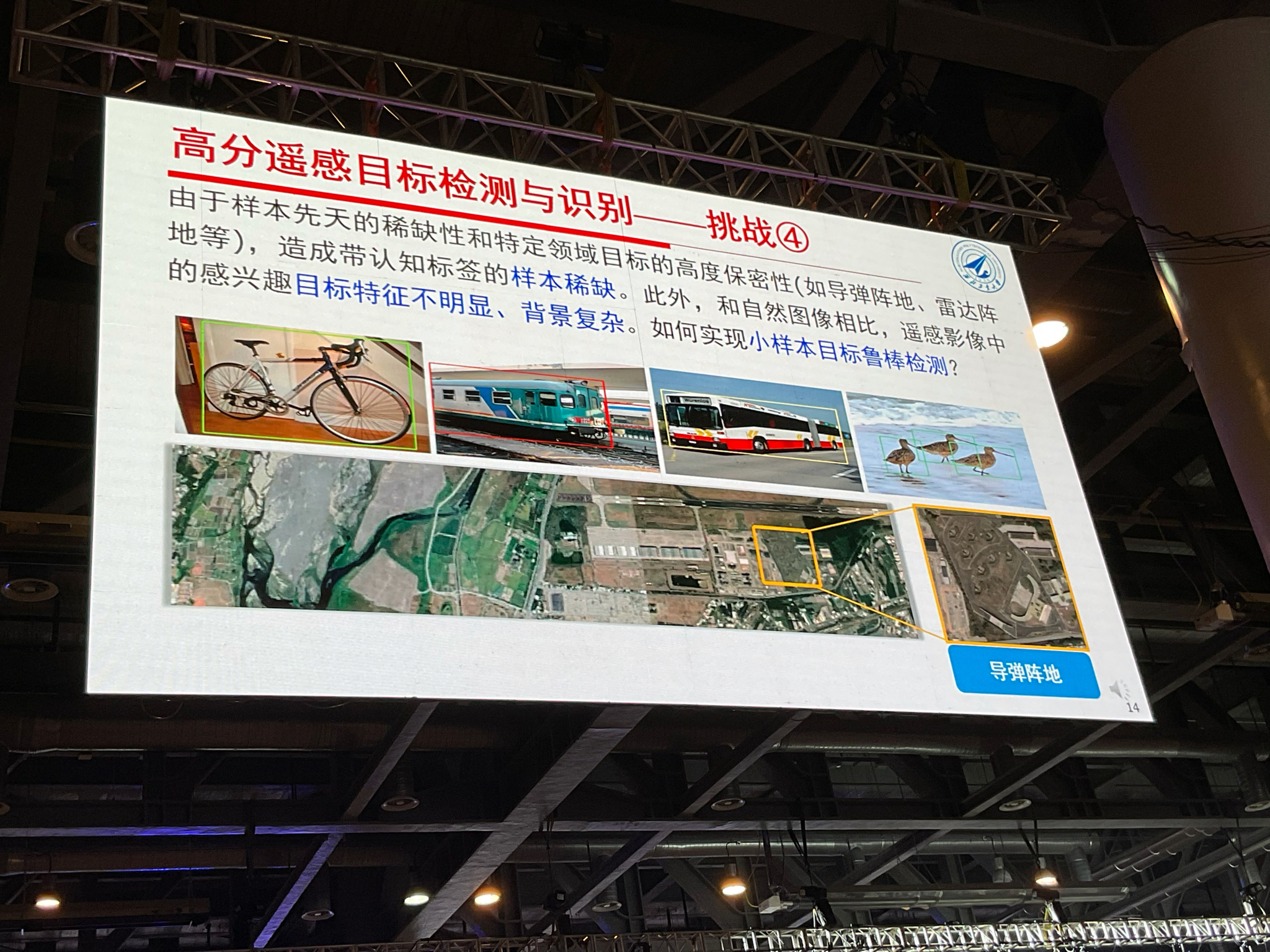
3.弱监督学习
4.小样本
Cheng G, Cai L, Lang C, et al. SPNet: Siamese-Prototype Network for Few-Shot Remote Sensing Image Scene Classification[J]. IEEE Transactions on Geoscience and Remote Sensing, 2021.
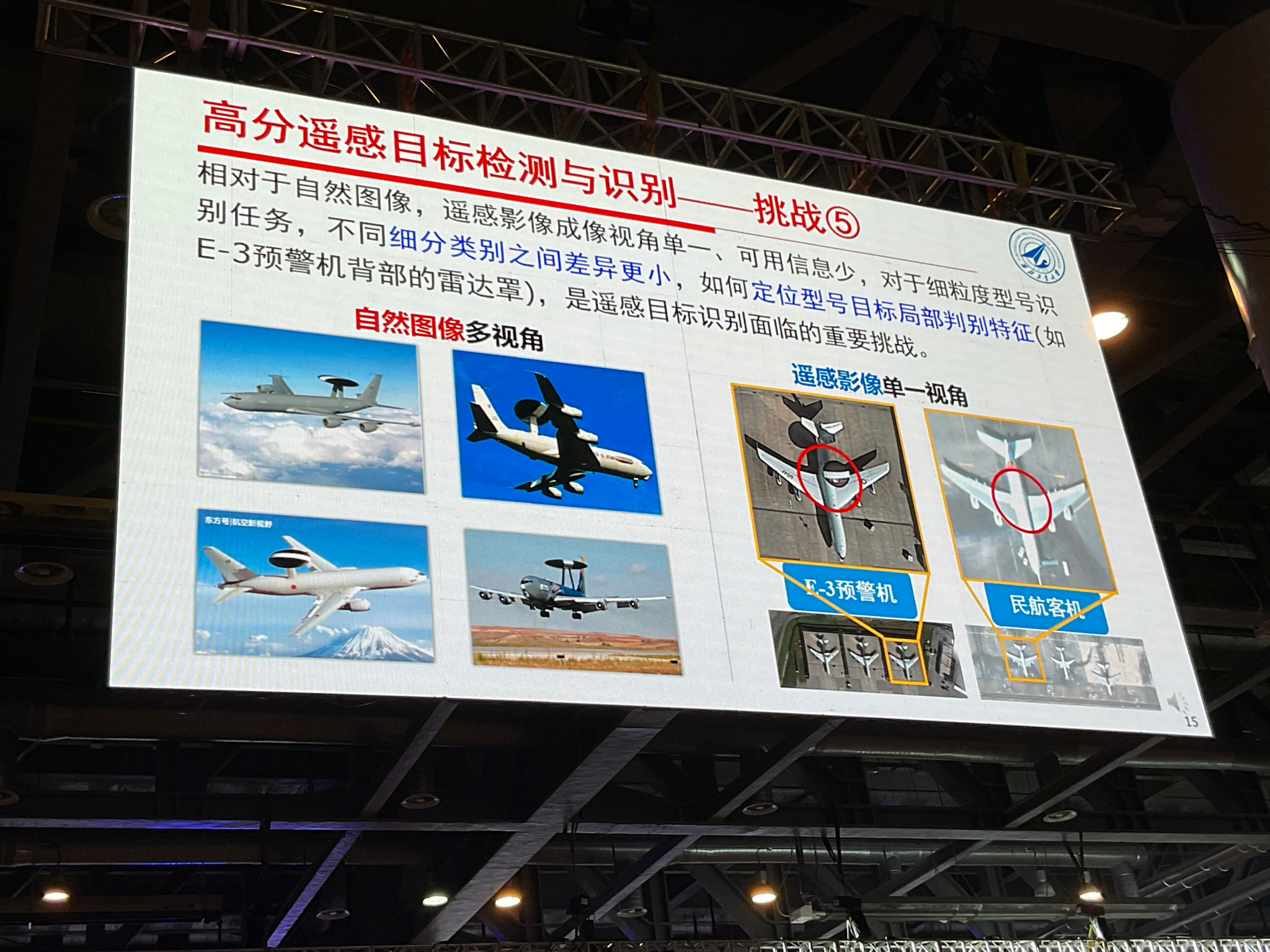
5.细粒度
Han J, Yao X, Cheng G, et al. P-CNN: Part-based convolutional neural networks for fine-grained visual categorization[J]. IEEE transactions on pattern analysis and machine intelligence, 2019.
6.研究背景及展望
![]() |
![]() |
- 快速、轻量、大幅度画面
- 弱小目标检测
- 域自适应
时间:10.09
模式识别:从分类到理解
![]() |
![]() |
![]() |
![]() |
时间:10.09
视频理解
具体不是很懂
不过发现Transformer在视频领域也得到了应用。
Arnab A, Dehghani M, Heigold G, et al. Vivit: A video vision transformer[J]. arXiv preprint arXiv:2103.15691, 2021.
时间:10.09
分布外(Out-Of-Distribution)泛化
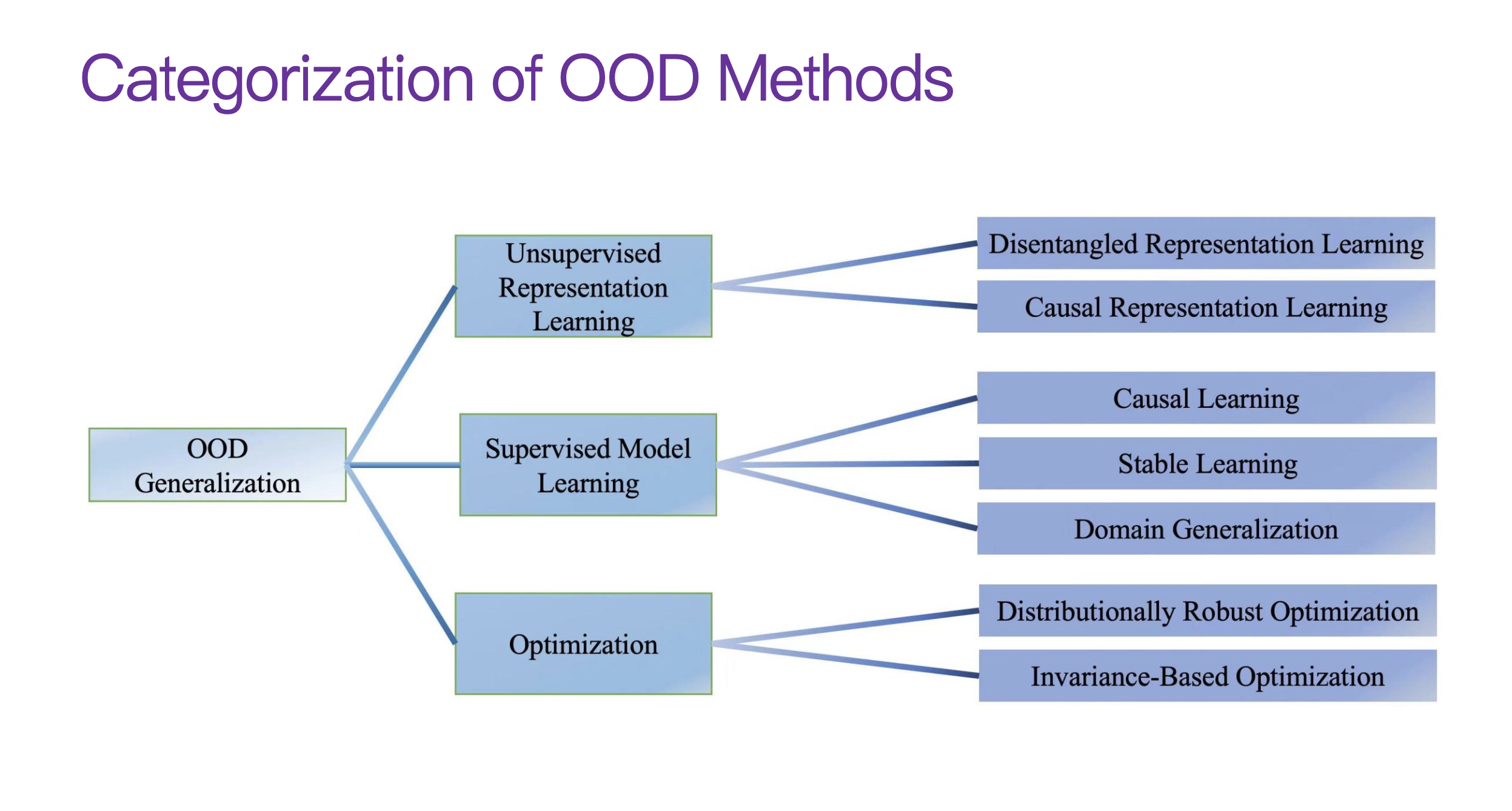
Shen Z, Liu J, He Y, et al. Towards Out-Of-Distribution Generalization: A Survey[J]. arXiv preprint arXiv:2108.13624, 2021.[PPT]
此外崔老师还做了一个因果推断的Tutorial[PPT]
时间:10.09
对抗视觉生成与合成年度进展概述
关于GAN的发展历程
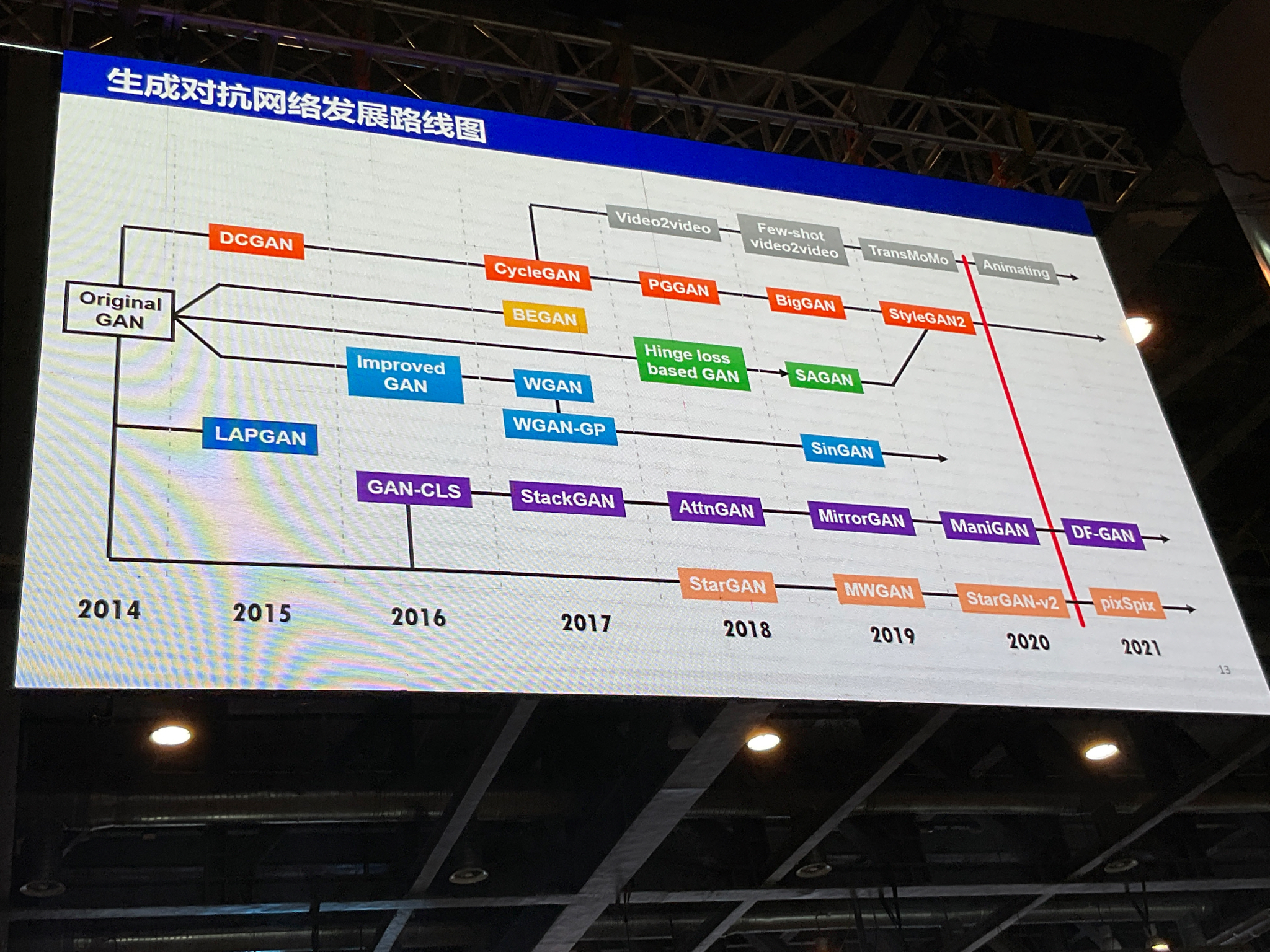
Pixel2style2pixel
Richardson E, Alaluf Y, Patashnik O, et al. Encoding in style: a stylegan encoder for image-to-image translation[C]//Proceedings of the IEEE/CVF Conference on Computer Vision and Pattern Recognition. 2021: 2287-2296.
![]() |
![]() |
时间:10.09
基于物理模型的计算光谱摄像
正向成像模型
$Y=H(X)+N$
计算重构模型
$\hat{X}=argmin \left | Y-H(X)\right |^{2}_{2}+\lambda\Phi(X)$
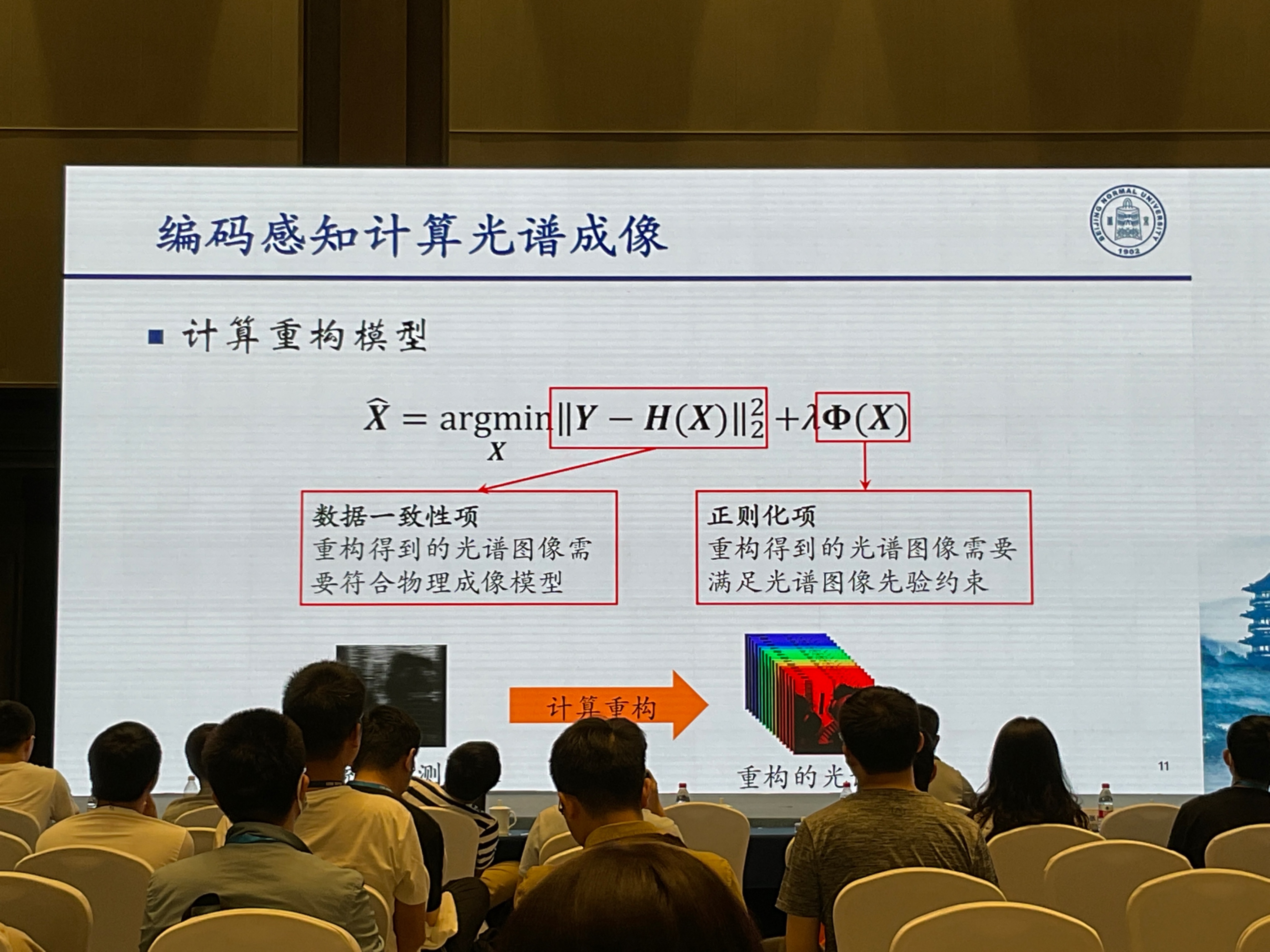
要构建基本模型+数据驱动的深度学习方法
![]() |
![]() |
![]() |
![]() |
时间:10.10
Normalization
之前从未关注过,有关Normalization的研究。作为深度学习模型中重要的一环,值得花时间深入理解一下。
- 什么是Normalization?
- 使用Normlization的动机是什么?
![]() |
![]() |
![]() |
![]() |
时间:10.10
全生命周期的自动化机器学习算法研究和行业实践
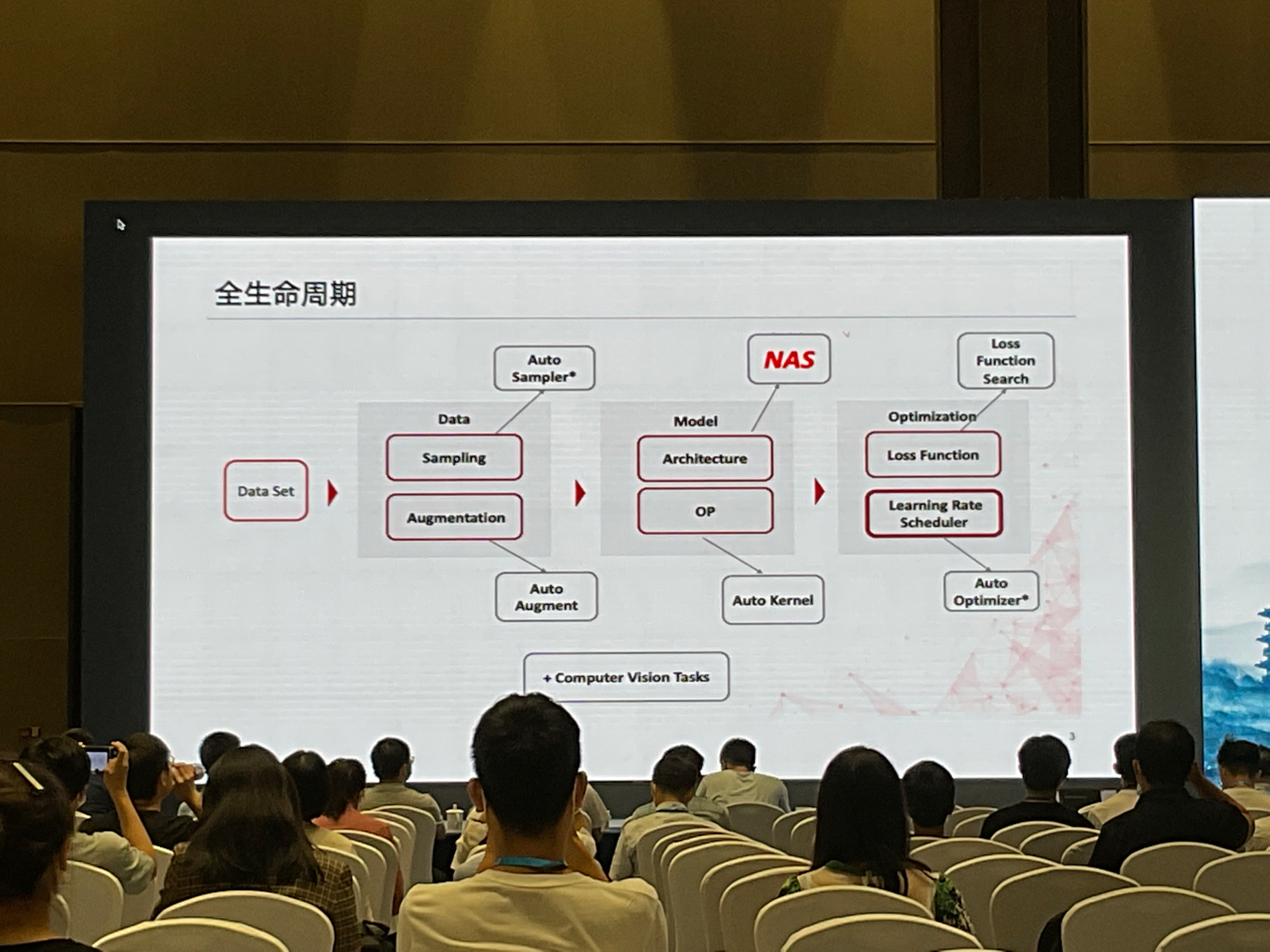
时间:10.10
视觉基础模型架构设计新思路
不得不佩服大佬的思路,大佬是怎么思考ViT的。
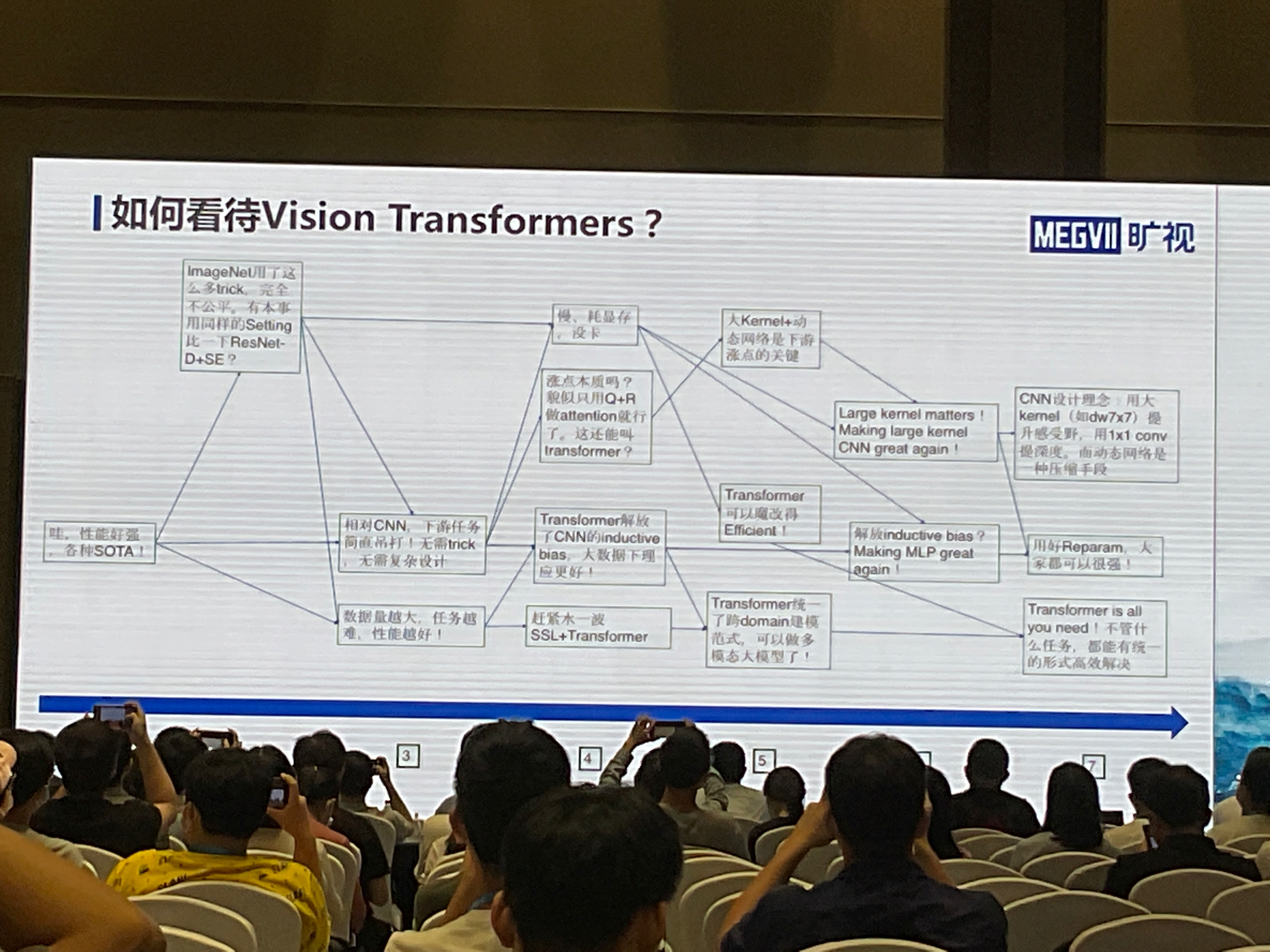
其中关键在与大感受野(大卷积核)Muti-head Self-Attention不是必须的。
![]() |
![]() |